TEXT DATA ANNOTATION SERVICES
Text Data Annotation Services by Pangeanic: a customized solution. Elevate Your Machine Learning Models
Looking for top-tier text data annotation solutions? Pangeanic offers tailored solutions for all your data annotation needs. Enhance your machine learning models with high-quality text data annotation services
Text Data Annotation Use Cases
Our multilingual text data team has offered text data annotation services in over 100 languages, dialects and linguistic variants!
Symanto
Data annotation for information extraction on cryptocurrency from social media inputs, articles and websites.
NLPC
An NLP and data-for-ai company
Projects include Text annotation services from users for eCommerce purposes, hate speech severity, and tagging relevant information on mobile phone companies.
What makes us different?
We are developers of Natural Language Solutions. We used to be a language services company. We found that marrying both skills, our Data Department could offer text data annotation services for our government-funded research projects and to help other organizations improve their AI and specific Machine Learning projects.
Pangeanic has added our expertise in human-in-the-loop (HITL) quality control. Our PECAT tool allows for human review of machine-generated annotations to ensure the highest quality.
“We understand each client is different, each project is different and many projects are very specific. Our customized solutions make all the difference: PECAT is so flexible that it can be tailored to meet your specific labeling needs and requirements.”
Amando Estela - VP of Revenue
Discover its features:
Quality AI training
Equip your AI systems with the best training data.
Accurate and relevant results
Benefit from results that matter and are relevant to your needs.
Monolingual & Multilingual Annotation
Cater to a global audience with diverse linguistic support.
Professional Reviewing
Enhance data quality with Human-in-the-loop oversight.
Versatile PECAT Tool
Supports diverse user profiles for varied annotation requirements.
PECAT: Our advanced text data annotation tool
Pangeanic’s proprietary tool, PECAT, not only facilitates monolingual and multilingual data labeling, but also integrates all the features you might expect from an NLP team that understands your needs: glossaries and regex for enhanced data labeling accuracy, access to LLMs or even your own Pre-labeling tools. Our experienced annotators ensure accurate and relevant results, while our PECAT tool provides advanced features for multilingual annotation and human-in-the-loop quality control.
-
Support for monolingual and multilingual databases
-
Glossaries and regular expressions
-
Human-in-the-loop capabilities
-
Quality control reports
Unlock the power of your data with text annotation
Text data annotation is a critical step in the development of machine learning models. By labeling data with relevant information, you can help your models understand the nuances of human language and improve their ability to perform Natural language processing (NLP) and AI applications such as:
-
How does text data annotation help sentiment analysis?
Text data annotation plays a pivotal role in improving the accuracy and reliability of sentiment analysis models, for example:
- Training Data Creation: Machine learning models need a considerable amount of annotated data to understand positive, negative and even complex and nuanced sentiments in texts. Human annotators label texts as ‘positive’, ‘negative’, ‘neutral’, or even with more nuanced emotions like ‘anger’, ‘joy’, or ‘sadness’. This labeled data serves as the foundation for training sentiment analysis models.
- Disambiguation: Context is always crucial in sentiment analysis. For instance, the word “sick” can mean “ill” or, in slang, “impressive”. Human annotators can understand such nuances and annotate text accordingly, helping models to differentiate based on context and thus come closer to human understanding.
- Improved Model Accuracy: As models are trained on human-annotated data, their prediction accuracy for new, unseen data improves. The clearer and more precise the annotations, the better the model becomes at sentiment detection.
- Handling Sarcasm and Idioms: Sarcasm are a very human and ad-hoc means of communication. Idioms are extremely challenging for algorithms to detect as well because they read as a natural expression but their meaning is based on a cultural setting and tradition (that’s why idioms are so difficult to translate). With annotated data highlighting these subtle linguistic features, models can be trained to recognize typical sarcastic expressions and idiomatic expressions and correctly interpret them.
- Support for Multiple Languages: Obviously, text data annotation can be done for various languages, enabling sentiment analysis tools to work effectively across different languages and cultures, as we’ve just mentioned for the cases of sarcasms or idioms which may or not have an equivalent in another language but that surely mean nothing if taken literally (for example the German “Da brat mir doch einer einen Storch” literally means “Someone is roasting/frying me a stork” is a set expression often used when someone is very surprised something [very unlikely to happen] actually happened)
- Continuous Learning: As language evolves and new expressions or slangs emerge, annotated data can be updated to include these changes, ensuring that sentiment analysis models remain current.
- Customization for Specific Domains: Different industries may have unique jargons or ways of expressing sentiment. By annotating text data specific to a domain (e.g., medical, financial, or technical), sentiment analysis models can be finely tuned for that domain.
-
How does text data annotation help information extraction?
Text data annotation can aid information extraction by identifying specific events or incidents mentioned in a text and annotating them accordingly. This annotation type helps in information extraction, news analysis, and event monitoring. By labeling events, researchers and analysts can detect patterns, track trends, and gather insights from textual data related to real-world occurrences. Additionally, dependency parsing, which annotates text by identifying the grammatical relationships between words in a sentence, can also support information extraction. Text annotation provides the necessary foundation for transforming unstructured text into structured and actionable data, facilitating knowledge graph construction and powerful search and recommendation systems.
-
Identifying and labeling entities: Text data annotation can be used to identify and label entities in text, such as people, places, organizations, dates, and events. This information can then be used to extract structured data from unstructured text. This can be done manually or using automated tools. Once entities have been labeled, they can be used to extract information from text. For example, if you have a dataset of news articles, you could use text data annotation to identify the names of people, organizations, and places mentioned in the articles. This information could then be used to create a database of people, organizations, and places.
-
Identifying relationships between entities: Text data annotation can also be used to identify relationships between entities. For example, an annotator might identify that a particular person is the CEO of a particular company. This information can be used to create a knowledge graph that can be used to answer questions about the data.
-
Improving the accuracy of information extraction models: Text data annotation can be used to improve the accuracy of information extraction models. By providing models with high-quality training data, annotators can help them to learn to identify and extract information more accurately.
-
Reducing the time and effort required for information extraction: Text data annotation can help to reduce the time and effort required for information extraction. By providing models with pre-annotated data, annotators can free up human experts to focus on more complex tasks.
-
Extracting structured data from unstructured text: Text data annotation can be used to extract structured data from unstructured text. For example, you could use text data annotation to extract the date, time, and location of an event from a news article. This information could then be stored in a database.
-
Improving the accuracy of machine learning models: Text data annotation can be used to improve the accuracy of machine learning models. For example, you could use text data annotation to train a machine learning model to identify named entities in text. This model could then be used to identify named entities in new text.
-
-
How does text data annotation help in Question answering (QA)?
Text data annotation provides the foundational knowledge and context for QA systems. It helps them understand the intricacies of human questions and how to extract or formulate accurate answers from data sources. If you generate properly annotated data, you’ll ensure that QA systems respond effectively and accurately to user queries. In general, Question Answering (QA) systems aim to provide accurate answers to user queries based on a given text or a vast corpus of data. Text data annotation plays a crucial role in enhancing the performance of these systems.
-
Training Data Preparation: For machine learning-based QA systems, annotated datasets are essential. Annotators can label specific portions of text as answers to particular questions, enabling models to learn how to identify correct answers.
-
Identifying Answer Types: Questions can seek different types of answers: names, dates, numbers, locations, etc. Annotated data can specify the expected answer type, guiding the QA system in its response.
-
Contextual Understanding: Some answers depend heavily on context. Annotated datasets can help models discern nuances and contexts in which certain answers are relevant.
-
Handling Ambiguity: Questions can often be ambiguous. Annotations can clarify possible interpretations of a question and the corresponding appropriate answers.
-
Supporting Evidence Extraction**: For systems that not only provide answers but also evidence or reasoning behind the answer, annotated data can highlight supporting passages or facts.
-
Multi-turn Conversations: Advanced QA systems engage in multi-turn conversations where the context from previous questions is used in subsequent ones. Annotated dialogues can help models maintain and leverage context across a conversation.
-
Domain-Specific QA: Text data annotated for specific domains (e.g., medical, legal, technical) can train QA systems to understand and answer questions pertinent to that domain with higher accuracy.
-
Evaluation and Benchmarking: Annotated datasets can serve as a ground truth for evaluating the performance of QA systems, helping in benchmarking and further improvement.
-
Feedback Loop: As QA systems are used, user feedback can be integrated as annotations to refine and retrain the models, ensuring continuous learning and adaptation.
-
Handling Diverse Languages and Cultures: QA systems need to work across languages and cultures. Annotated data in various languages can help in training multilingual models, while cultural annotations can ensure that the system's responses are contextually and culturally appropriate.
-
-
How does text data annotation help in machine translation?
Text data annotation is vital for improving the performance and reliability of MT systems. Here’s how text data annotation assists in machine translation:
-
Training Parallel Corpora: The foundation of all statistical and neural machine translation systems is parallel corpora—texts (source language) and their corresponding translations (target language). Annotated datasets with source-target language pairs help in training models to understand translation equivalents.
-
Phrase Alignment: For phrase-based translation systems, annotations can highlight which phrases in the source language correspond to phrases in the target language, aiding in more accurate translation.
-
Handling Ambiguity: Many words have multiple meanings based on context. Annotated data can clarify the intended meaning in a given context, enabling the MT system to choose the correct translation.
-
Grammar and Syntax: Annotations can provide insights into the syntactic structures of sentences, helping translation models to generate grammatically correct outputs in the target language.
-
Cultural Context: Translation isn’t just about words—it’s also about conveying cultural context. Annotations can provide cultural notes or context clues, ensuring translations are culturally sensitive and appropriate.
-
Terminology Consistency: Especially in specialized fields like medicine or law, consistent terminology is crucial. Annotated datasets can help MT systems recognize and consistently translate domain-specific terms.
-
Evaluation Metrics: Annotated translation datasets can serve as a “gold standard” to evaluate the quality of machine translation outputs, using metrics like BLEU, TER, and others.
-
Feedback Loop: Post-editing annotations, where human translators correct machine-generated outputs, can be fed back into the MT system for continuous model refinement.
-
Handling Idioms and Colloquialisms: As we’ve mentioned above, the literal translation of idioms often doesn’t make sense in the target language. Annotations can highlight idiomatic expressions and suggest appropriate translations.
-
Morphological Information: Some languages are morphologically rich, meaning words can take on many forms. Annotations can provide information about the root forms, genders, cases, tenses, etc., assisting in more accurate translation.
-
Multimodal Translation: In tasks where translation relies not just on text but also on other modalities like images or video, annotations can link textual information with visual cues, improving translation relevance.
In essence, text data annotation acts as a guiding mechanism, enabling machine translation systems to navigate the complexities of languages, ensuring the outputs are not just linguistically accurate but also contextually and culturally appropriate. Properly annotated data is crucial for training robust and efficient MT systems.
Text data annotation helps in machine translation in a number of ways:
-
It provides training data for machine translation models. Machine translation models are trained on large amounts of parallel data, which consists of pairs of sentences in two languages. The model learns to translate text by identifying patterns in the parallel data. The more training data the model has, the better it will be able to translate text.
-
It helps to improve the accuracy of machine translation models. By identifying and correcting errors in training data, annotators can help to improve the accuracy of machine translation models. This is especially important for languages that are difficult to translate, such as those with complex grammar or a large number of homophones.
-
It helps to make machine translation models more adaptable to different types of text. By annotating text from a variety of genres and domains, annotators can help to make machine translation models more adaptable to different types of text. This is important for ensuring that machine translation models can be used to translate a wide range of content.
-
It helps to improve the fluency of machine translation output. By identifying and correcting unnatural or awkward phrasing, annotators can help to improve the fluency of machine translation output. This is important for ensuring that machine translation output is easy to read and understand.
In short, text data annotation is essential for developing high-quality machine translation models. By providing training data, improving accuracy, and enhancing fluency, annotators help to make machine translation a more powerful and versatile tool. Here are some specific examples of how text data annotation can be used to improve machine translation:
-
Annotating named entities can help machine translation models to correctly translate names of people, places, and organizations.
-
Annotating part-of-speech tags can help machine translation models to understand the grammatical structure of sentences.
-
Annotating semantic roles can help machine translation models to understand the meaning of words and phrases.
-
Annotating sentiment can help machine translation models to convey the emotional tone of text.
-
By annotating text with this type of information, annotators can help to improve the accuracy, fluency, and naturalness of machine translation output.
-
Pangeanic offers a wide range of text data annotation services to meet your specific needs.
Our experienced annotators are trained to provide high-quality results that are accurate, relevant, and consistent.
Our text data annotation services include:
Entity recognition
Named entity recognition (NER)
Part-of-speech (POS) tagging
Semantic role labeling
Coreference resolution
Sentiment analysis
Topic modeling
Intent classification
Question answering
Key Benefits of Pangeanic’s Data Annotation Services
With Pangeanic, your ML project will obtain high-quality results. Our annotators are trained to provide accurate, relevant, and consistent results in many text data annotation projects, from classification of cryptocurrency documents to sentiment analysis, hate speech detection and data labeling for LLMs. Because of our roots as a translation services company and a developer of machine translation systems since 2010, we have built a massive network of freelance linguists and language-aware data annotators to offer full multilingual support in all text data annotation projects.
Why Choose Pangeanic’s Text Annotation Solutions?:
Data annotation is pivotal in refining Machine Learning (ML) models. Through meticulous labeling and feature identification within datasets, AI systems are empowered to discern patterns more effectively. This translates to:
-
Recognizing customer intent in messages.
-
Unveiling insights from user search behaviors.
-
Elevating your content strategy with keyword extraction.
In addition to these direct benefits, text data annotation can also help to improve the overall quality of information extraction systems. By providing models with high-quality training data, annotators can help them to learn to identify and extract information more accurately. This can lead to improved performance on a wide range of information extraction tasks. For example, text data annotation can be used to improve the accuracy of named entity recognition (NER), which is the task of identifying and classifying named entities in text. NER is a critical component of many information extraction systems, and improving its accuracy can lead to improved performance on tasks such as information retrieval, question answering, and machine translation.
Other example of how text data annotation can be used in information extraction:
-
Customer relationship management (CRM) systems: CRM systems use text data annotation to extract information from customer interactions, such as emails, phone calls, and social media posts. This information can then be used to create a more complete picture of each customer.
-
Fraud detection systems: Fraud detection systems use text data annotation to identify fraudulent transactions. For example, a fraud detection system might use text data annotation to identify transactions that are associated with known fraudulent email addresses or phone numbers.
-
Medical research: Medical researchers use text data annotation to extract information from medical records. For example, a medical researcher might use text data annotation to extract information about a patient's symptoms, diagnoses, and treatments.
Text data annotation is a valuable tool for improving the performance of information extraction systems. By providing models with high-quality training data, annotators can help them to learn to identify and extract information more accurately.
Learn how to achieve your project objectives with Pangeanic
Over 20 years of experience
At the forefront of NLP technologies
Security and Privacy
ISO-certified, guaranteeing quality and secure workflows
Scalable solutions
Customized solutions to fit your needs
European Commission MAPA Project
The European Commission's MAPA project uses Pangeanic's Data Annotation services to label named entities with a high level of granularity (nested elements).
Why Choose Pangeanic’s Text Annotation Solutions?:
At Pangeanic, our goal is to propel your business forward. By synergizing cutting-edge AI with human expertise, we deliver tailored annotation services that let you harness the true power of technology.
If you are looking for a reliable and experienced text data annotation provider, Pangeanic can help. Contact us today to learn more about our services and how we can help you achieve your machine learning goals.
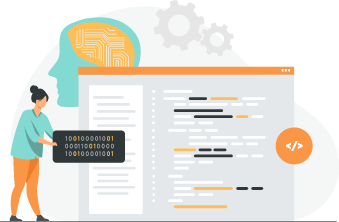