Pangeanic can help you with knowledge discovery
Imagine having a huge amount of data that needs to be processed in order to understand it, or Big Data sets that need to be processed every day. This data is obtained from different inputs. Many of these are documents, but there are also emails, voice recordings, text-based news clips, radio interviews, websites, third-party documentation in PDF format, scanned images and text, videos, etc.
You may also receive key financial information from various banks and financial institutions, from which you only need to collect key information such as names of individuals, names of institutions, or exchange rates between different currencies. Pangeanic's AI solutions identify the right data quickly so you can take the best decisions.
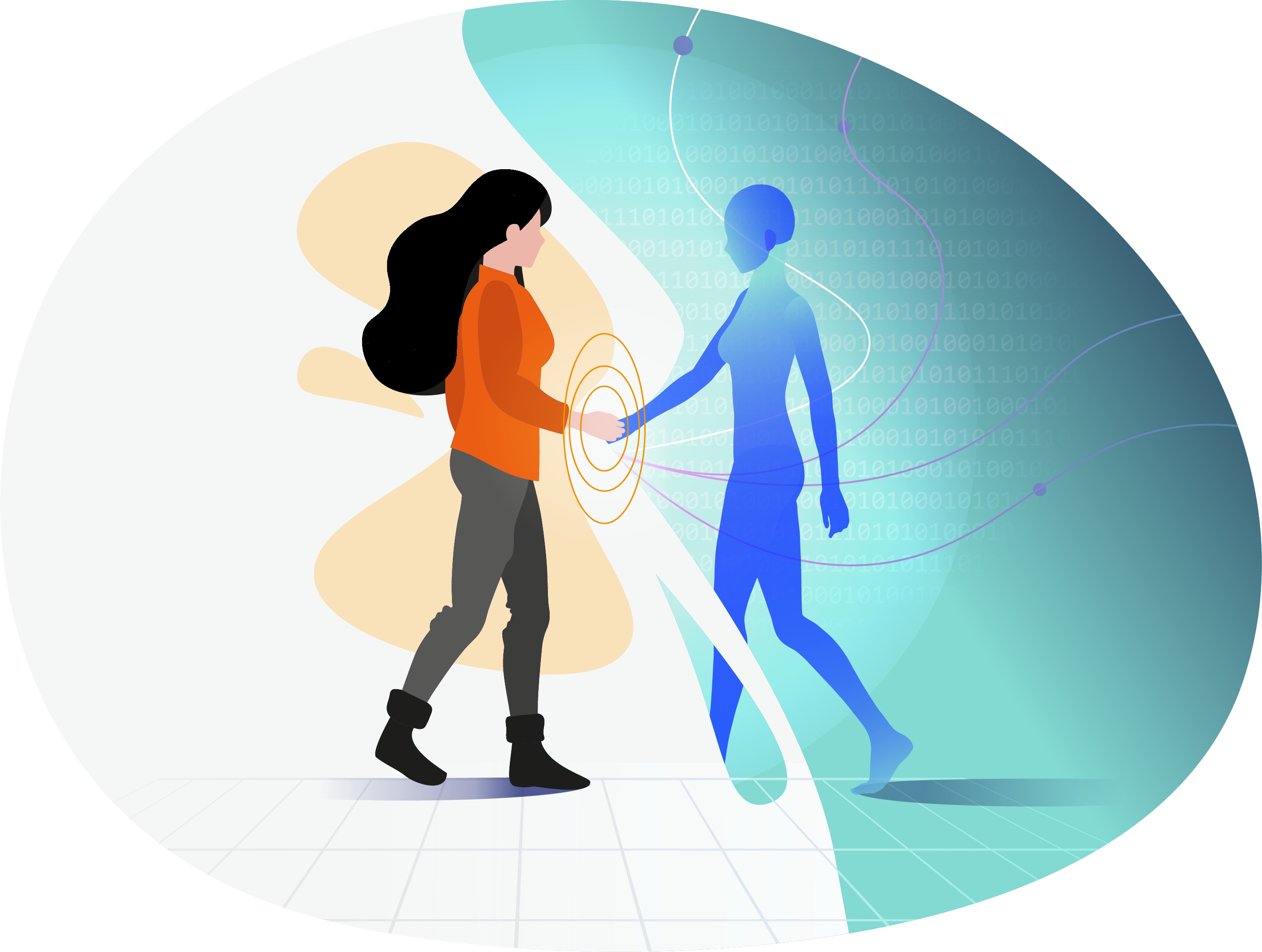
Typical use cases of Knowledge Engineering and Discovery
Creating csv/spreadsheets with financial data from central banks and financial institutions on currency forecasts
Providing a summary report on a topic by extracting data from a variety of sources
Labeling Big Data with typical NLP labels for Machine Learning
Extracting names, places, and actions from a series of television programs, internet videos, or radio interviews, using speech-to-text for extraction
Finding feedback on social media posts and tagging each tweet or comment as positive or negative
Automatically sorting documents according to a predefined field and creating an overview
Knowledge Engineering and Discovery tools
Pangeanic's Knowledge Engineering and Discovery tool is here to do the work for you with the utmost precision: no matter the source, we will convert it into text so it can be processed. Through a series of NLP techniques, we structure the data so that key information can be extracted for you in a user-friendly format. It can be a list of actions, keywords, quantities extracted from tables of different forms, key phrases or a complete labeling of the material so that all kinds of actionable information can be extracted.
Knowledge Discovery (K-Discovery) should not be confused with e-Discovery, as it goes further than extracting information from a given list of keywords while leaving the source intact. K-Discovery provides structure to the source text so that Machine Learning and Data Mining methods can be applied for any type of structured information retrieval at a later stage. It differs from Summarization because it does not aim to create an abstract representation of meaning for rapid processing by humans (which may be an end use of Knowledge Discovery).
It applies knowledge to the source, meaning many types of actions can be performed, and several types of uses can be derived from it.